
How did we get here? A short history of artificial intelligence
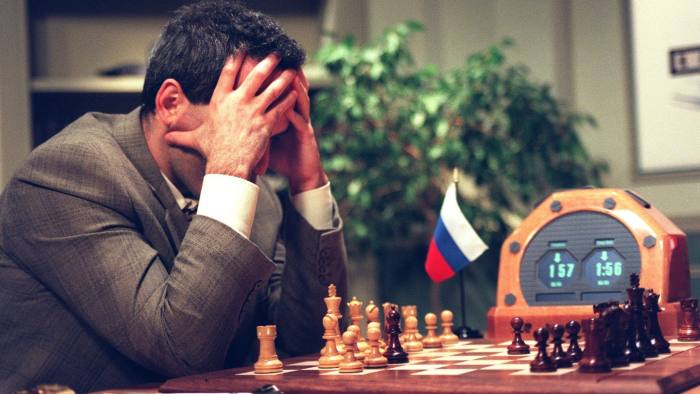
For over seventy years the story of artificial intelligence (AI) has been one of saturating the capabilities of AI to the level of computational power available, and then waiting for Moore’s Law to catch up. During that time there have been bubbles of AI hype, research booms, funding busts and a quiet rebirth. Here we explore a short history of AI:
Alan Turing
The AI story starts with Alan Turing, a young British polymath who explored the mathematical possibility of artificial intelligence. Turing suggested that humans use available information as well as reason in order to solve problems and to make decisions. Turing postulated that machines which could do the same thing.
This was the logical framework for Turing’s 1950 paper ‘Computing Machinery and Intelligence’ in which he discussed how to build intelligent machines, as well as how to test their intelligence.
Early limitations
Before 1949 computers lacked a key prerequisite for artificial intelligence: memory. Computers could not store commands, only execute them. They could be told what to do, but they could not then remember what they did, and they certainly couldn’t learn.
Computing was also extremely expensive in the early 1950’s. Only prestigious universities and big technology companies could afford to experiment with them. There was no ‘proof of concept’ that research and development into the possibility of artificial intelligence was something that was worth investing in.
The Logic Theorist
The Logic Theorist was a program designed to mimic the problem-solving skills of a human. It was presented in 1956 at the Dartmouth Summer Research Project on Artificial Intelligence. The conference brought together top researchers from various fields for an open-ended discussion on artificial intelligence. The term ‘artificial intelligence’ was coined at the event, which became a catalyst for the next twenty years of AI research.
The AI ‘boom and bust’ of 1957 – 1974
AI flourished between 1957 and 1974 as computers that could store ever-increasing quantities of information became faster and cheaper. Machine learning algorithms also improved as researchers started to understand their power; and DARPA began to fund AI research at several academic institutions in the USA.
‘AI optimism’ grew into a bubble of high expectation that peaked in 1970 when Marvin Minsky told Life Magazine that: “within three to eight years we will have a machine with the general intelligence of an average human being.” In reality it would still be decades before many of the key components of AI would become a reality.
By the end of the 1970’s it was clear that AI had been over-hyped. Computers still couldn’t store enough information or process it fast enough for ‘workable’ AI. In the absence of natural language processing computers had no way of understanding the intended meaning of different combinations of words. As the world realised that computers were still millions of times too weak to exhibit intelligence, most sources of AI research funding dried up.
The re-birth of AI in the 1980’s
In the 1980’s, interest in AI was reignited. John Hopfield and David Rumelhart popularized “deep learning” techniques that allowed computers to learn from experience. Edward Feigenbaum introduced expert systems that mimicked the decision-making process of a human expert, which became widely used in industry.
Amidst its’ technology-driven economic hyper-growth of the 1980’s, the Japanese government heavily funded AI innovations. Between 1982 and 1990 they invested $400 million dollars with the goals of revolutionizing computer processing, implementing logic programming, and improving artificial intelligence.
Most of these goals were not met by 1990, but the projects inspired a talented generation of engineers and scientists to pursue AI.
The deceptive decade: AI in the 1990’s
Most disruptive exponential growth trends go through a ‘deceptive’ phase where their doubling or tripling in size each year goes unnoticed because it starts from such a small base. AI spent the 1990’s in this ‘deceptive’ phase, when in the absence of hype, AI quietly thrived.
Many of the landmark goals of artificial intelligence were achieved during 1990s and early 2000s. In 1997, reigning world chess champion and grand master Gary Kasparov was defeated by IBM’s Deep Blue, a chess playing computer program. In the same year, speech recognition software was implemented in Windows.
Suddenly it seemed as though there wasn’t a problem that machines couldn’t handle. Machines even started to learn human emotion with the advent of Kismet, a robot that could recognize and also display emotions.
Moore’s Law
Scientists and researchers didn’t get smarter about how they coded AI over the last few years. It was mostly the limits of computer storage that for decades restrained their ability to create ‘real’ AI.
During the last 30 years Moore’s Law, which estimates that the memory and speed of computers will double every year, has not just become manifest but has exceeded its own predictions. This is how Deep Blue was able to defeat Gary Kasparov in 1997, and how Google’s Alpha Go was able to defeat Chinese Go champion, Ke Jie, in 2016.
When it comes to AI research, we saturate the capabilities of AI to the level of our current computational power (computer storage and processing speed), and then we wait for Moore’s Law to catch up again. That in many ways is what summarises the history of AI.
Where does AI go next?
There may be evidence that Moore’s law is slightly slowing down, but the increase in data certainly hasn’t lost any momentum. Breakthroughs in computer science, mathematics and neuroscience all serve as potential new avenues of AI innovation that will continue to break through the ‘ceiling’ of Moore’s Law.
That we now live in an age of ‘big data’, in which we have the capacity to collect huge data sets of information that are too cumbersome for a human to process, is also relevant. Even if the growth in processing power slows and algorithms do not improve much, feeding AI with ‘even bigger’ data allows it to learn through ‘brute force’ and therefore continue to optimise and improve.
The long-term goal for AI is general intelligence. That is a machine which surpasses human cognitive abilities in all tasks. That would be a machine along the lines of the sentient robots that we are used to seeing in movies. It seems inconceivable that this could be accomplished in the next decade. But within fifty years, given the progress made since 1949? Maybe.